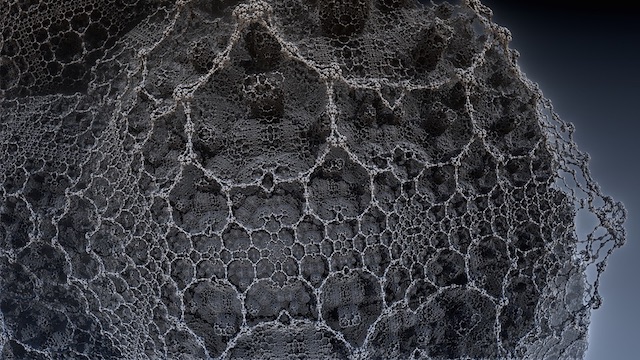
Scientists from the University of Michigan have created a new way to teach machines. This technology will allow machinery to learn and think much the way that human beings do. The neural network relies on memristors; it was able to predict words before they were said during a conversation. It was also capable of predicting future events by extrapolating from current situations.
Memristors are a resistive device capable of performing logic and storing data. They are fundamental to the success of the new network, which is called a reservoir computing system. The reservoir computing system differs from previous networks by reducing the training time necessary for success. Additionally, the use of memristors cut down on the need for space. They are also far easier to integrate with existing silicon-based electronics. Traditional computer systems require memory modules, which are kept separate from the processors that perform logic. This requires significantly more space than memristors, and is ultimately a less effective system.
Neural networks are designed to imitate an organic living brain. They are made up of neurons (or nodes). Synapses provide the connections between the nodes. Scientists provide the neural network with a large set of questions, as well as answers to the questions. Connections between nodes are weighted more or less, which reduces the amount of error in finding the correct answer. This process is called supervised learning.
After it has been effectively trained, a neural network can be tested. This time, answers to the questions are not provided. Unfortunately, it’s no easy feat getting the machines to this point; it can take months of work and a lot of money to thoroughly train the technology.
That’s where the memristors make a dramatic difference. If they’re built using memristors, reservoir computing systems simply don’t require a lengthy and expensive training process. The reservoir component is capable of identifying important time-related features of any inputted data. It then passes said data along to a second network, in a simpler format. While the second network does require training, it is of a far simpler variety.
The end result is a computer network capable of a variety of impressive feats. It was able to pass a test of handwriting recognition with 91% accuracy. The network also proved itself capable of handling data that changes over time, and predicting what will come next. Researchers plan to explore the possibilities of speech recognition, as well as predictive analysis.