Statistical models for maximum electromagnetic fields within RF reflective enclosures (i.e. complex cavities) have historically been derived from parent distributions obtained from the central limit theorem applied to sums of random variables. However, recent work has been done to apply the Fisher-Tippett theorem and its associated generalized extreme value (GEV) distribution to model enclosure maximums directly. The purpose of this paper is to experimentally validate the GEV distribution to model maximum received power measurements within a complex cavity for use in Hazards of Electromagnetic Radiation to Ordnance (HERO) and Electromagnetic Vulnerability (EMV) testing. Distribution parameters and associated confidence intervals are statistically determined through maximum likelihood estimation (MLE) methods.
Electromagnetic fields within RF reflective enclosures are of interest since most electronic systems/subsystems are contained within metallic cavities of varying shapes and sizes. The enclosure fields are characterized by the complex interaction of many excited high-order modal field distributions that are very sensitive to small changes in boundary conditions, such as occur from changes in physical structure (mechanical mode stirring), frequency (frequency mode stirring), and incident energy points of entry (source mode stirring) [1].
A deterministic description, either by measurement or by modeling/simulation, of such ‘chaotic’ fields is neither practical nor useful, as changes are constantly occurring. Useful descriptions must be statistical in nature and independent of every minute detail about the space. Such statistical electromagnetic formalisms have been developed for the complex cavities that comprise reverberation chambers used in EMI/EMC testing [2].
The existing maximum value distribution functions in current complex cavity literature [1]-[5] are derived from parent distributions obtained from the central limit theorem applied to sums of random variables. This process leads to a maximum value distribution function that is formally unbounded, a physically unrealistic situation given the finite power density in the enclosure, and requires the cavity to be electrically large (or overmoded). However, an alternative statistical treatment utilizing maximum value extraction has been proposed to directly model the maximum fields within a cavity [6]. This approach utilizes the Fisher-Tippett theorem [7], which provides three possible asymptotic forms for the maximum value distribution (one of which is upper bounded) without requiring a priori knowledge of a parent distribution function. Therefore, by modelling only the extreme values (i.e. sample maximums), the distribution function is no longer limited to the overmoded case and provides a potential upper bounded distribution function.
In Hazards of Electromagnetic Radiation to Ordnance (HERO) and Electromagnetic Vulnerability (EMV) testing, maximum values are specifically of interest since all components within the enclosure will experience maximum fields at some point in time due to mode stirring. Additionally, prescribed test electromagnetic environments are typically defined by maximum (or peak) fields. It is therefore the distribution of those maximums with which we are most concerned.
The purpose of this paper is to build upon the work done by Orjubin [6] by experimentally validating the use of the generalized extreme value (GEV) distribution for maximum received powers within a complex cavity. The outline of the paper is as follows. In Section II, extreme value statistics are discussed including the Fisher-Tippett theorem, GEV distribution and parameter estimation techniques, followed by a discussion of the experimental design and approach in Section III. Section IV provides the experiments results including parameter estimates, confidence intervals, and probability plots.
Extreme Value Statistics
Fisher-Tippett Theorem & GEV Distribution
The Fisher-Tippett theorem states that for a large number of sample maximums derived from the same parent distribution, the cumulative distribution function (CDF) of these maximums will converge to one of three families of distributions (I, II or III) regardless of the parent distribution [7]:
(1)
where b, a, and α represent the location, scale and shape parameters and w represents the sample maximum values. Therefore, similar to how the probability density function (PDF) for sample averages converges to a normal distribution by the central limit theorem, the CDF of the maximums of any common parent distribution will converge to one of three extreme value distributions. These distributions are more commonly known as the Gumbel, Fréchet and Reverse Weibull distributions (I, II & III, respectively). The Fisher-Tippett theorem is therefore essentially the extreme value analog of the central limit theorem [7].
The identifying feature that distinguishes the three extreme value distributions is that they have significantly different high-end tail behavior. The Reverse Weibull distribution is asymptotically upper bounded, while the Gumbel and Fréchet distributions are unbounded and demonstrate exponential and polynomial asymptotic convergence, respectively. Therefore, determining the appropriate distribution function for a set of maximums is critical to ensuring low uncertainties for predicted extreme value behavior. However, by reformulating the three models into one distribution, specifically the GEV distribution, statistical implementation and distribution function selection is greatly simplified [7]. The GEV is then defined as:
(2)
where -∞ < m < ∞, s > 0, -∞ < k < ∞ and G is defined on w :
The model again is a multiple parameter distribution with location (m), scale (s) and shape (k) parameters. In fitting to the GEV, the tail of the distribution will be evident based on simple inference of the shape parameter, with k = 0 (Gumbel), k > 0 (Fréchet) and k < 0 (Reverse Weibull). It is important to note that specifically for the Gumbel model, a subset of the GEV family is interpreted as the limit of (2) as k → 0.
Parameter Estimation & Confidence Levels
There are various techniques for parameter estimation including nonlinear regression, moment-based methods, etc. each having their advantages and disadvantages. In this paper we utilize maximum likelihood estimation (MLE) due to standard and widely applicable approximations being available for standard deviation and confidence interval calculations. This method assigns different probability densities to the observed data for specific values of the parameters m, s, and k. The probability of the observed data as a function of the parameters is then termed the likelihood function. Typically, the log-likelihood function is utilized due to the convenience of summing values and since the logarithm function is monotonic, maximums will occur at the same point. The log-likelihood function for the GEV distribution is given by:
(3)
requiring for i = 1, …, N where N is the number of sample maximums.
Therefore, the estimated parameters are the values which maximize the log-likelihood function within its respective bounds. For complicated functions, numerical techniques are typically employed to ensure the global maximum is identified.
By defining θ as a multidimensional parameter consisting of the location, scale and shape parameters (m, s, and k), then for large N the maximum likelihood estimate asymptotically approaches a multivariate normal distribution (MVN) with:
(4)
where θ is the mean and Vθ is a 3×3 variance-covariance matrix. Therefore, confidence intervals for the individual components of can be determined based on the diagonal variance terms from the variance-covariance matrix.
Being that we are interested not only in the GEV distribution parameters (and their associated confidence intervals), but more importantly the specific probabilities of the extreme values, quantiles are advantageous to work with. By inverting (2) and defining and G(w) ≡ p and yp = −ln(p), the maximum likelihood estimate of (for 0 < p < 1) is then calculated as:
(5)
Then by theorem [7, pg. 33], the maximum likelihood estimate of any function of θ can be obtained by simple substitution of the estimation parameters into the respective function. Additionally, by the delta method, the maximum likelihood estimator of any scalar function of θ (specifically p) is normally distributed as:
(6)
with variance Vwp
(7)
Vθ is the variance-covariance matrix and ∇wp is the vector gradient of wp evaluated at . Therefore, the maximum likelihood estimate for the sample maximum
p and its associated 95% confidence intervals can be determined from (5) and (7) respectively.
Specifically in the k < 0 case (Reverse Weibull), inferences on the upper bound of the distribution can be made (effectively the as p → 1) and the maximum likelihood estimate for the upper bound
ub is defined as:
(8)
Experimental Design & Approach
Received power measurements were recorded within an RF reflective enclosure of dimensions 1.8 x 0.8 x 1.2 meters while being excited through a rectangular aperture of size 9.5 x 20 cm. Figure 1 depicts the general experimental configuration with the transmit antenna at a 2 meter distance from the aperture and a small dipole antenna inside the enclosure utilized for received power measurements. S21 measurements were recorded with a vector network analyzer from 7.55 – 7.65 GHz and an RF amplifier was used on the transmit path to ensure adequate dynamic range. Mechanical and frequency mode stirring, as well as spatial sampling, were all utilized for the experiment. Mechanical mode stirring was accomplished by stepping a metallic tuner located within the enclosure to 50 independent paddle wheel locations, while frequency mode stirring was performed over a 100 MHz frequency band. One hundred individual frequency measurements were recorded over the respective frequency band, leading to a ∆f equal to 1 MHz. This is much greater than the bandwidth in the cavity over which two adjacent measurements are correlated (i.e. the coherence bandwidth) in the frequency range of interest, thereby ensuring each sample was independent. Figure 2 provides photographs of the test enclosure and experimental configuration.
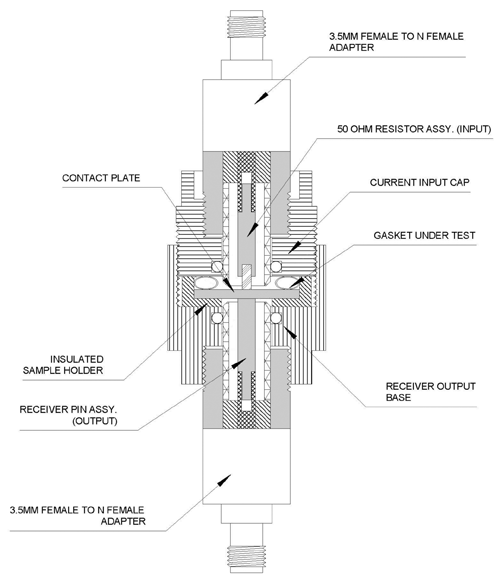
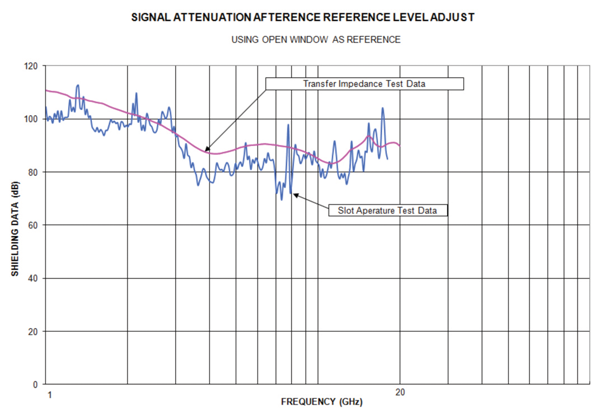
Each sample maximum was determined from the one hundred independent frequency samples for a single tuner position and receive probe location. Fifty tuner positions were performed for ten separate probe locations, producing five hundred sample maximums.
Experimental Results
Parameters & Variance-Covariance Matrix
The five hundred individual sample maximums w (i.e. maximum S21 measurements) are provided in Figure 3 and were statistically investigated in mean normalized linear units. Maximization of the GEV log-likelihood function (3) for this data set leads to the parameter estimates and variance-covariance matrix listed in Table 1. The diagonals of the matrix correspond to the variances of the individual parameters (m, s, k) while the off-diagonal terms indicate the covariances between respective parameters. A nonlinear regression tool was leveraged to determine initial input parameter estimates for the Matlab maximum likelihood estimate function (mle).
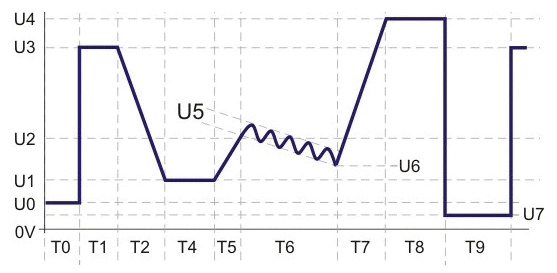
m |
s |
k |
|
Parameters |
.89 |
.20 |
−.035 |
Var−Cov Matrix |
m |
s |
k |
m |
9.8 ∙ 10−5 |
2.2 ∙ 10−5 |
-9.4 ∙ 10−5 |
s |
2.2 ∙ 10−5 |
4.9 ∙ 10−5 |
-5.6 ∙ 10−5 |
k |
-9.4 ∙ 10−5 |
-5.6 ∙ 10−5 |
8.4 ∙ 10−4 |
Table 1: GEV parameter estimates & variance covariance matrix
The shape parameter (k) was estimated to be less than zero indicating the appropriate model for the enclosure received power maximums is the Reverse Weibull GEV distribution (corresponding to the upper bounded distribution function). By combining estimates with standard errors, the approximate 95% confidence intervals for each parameter are [0.87, 0.91] for m, [0.19, 0.21] for s and [-0.09, 0.02] for k. Therefore, although the maximum likelihood estimate for k is negative, the 95% confidence interval does extend above zero, indicating that the data does not exclusively fit a bounded distribution function.
Model Diagnostics
There are various techniques for checking the validity of a model with respect to the measured data. Specifically, in the case of maximum distribution functions, uncertainties in the model parameters can lead to significant variation in the high-end tails of the distributions. Multiple diagnostic plots were investigated for the GEV model consisting of cumulative distribution functions (cdfs), quantile plots and quantile estimation plots, each based on a comparison of empirical data and the GEV distribution function. The three plots provide different insights into the validity of the model and the respective parameter estimates.
The cdf plot is a comparison of the empirical and fitted distribution functions and has been widely used in complex cavity literature as a “visual” validation tool for model goodness of fit diagnosis. The GEV cdf is depicted in Figure 4, showing very good agreement between the theoretical distribution (utilizing the estimated parameters in Table 1) and the ordered empirical data.
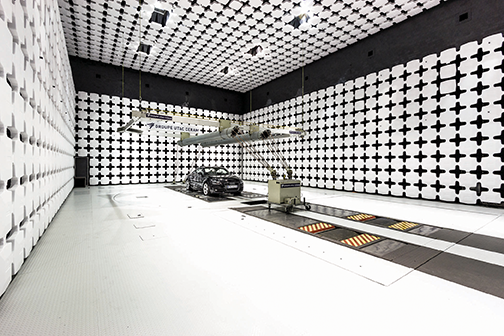
The quantile plot is a comparison of the empirical and estimated i/(n + 1) quantiles. This form of probability plot rescales the data to provide more information into the goodness of fit for the model at the high-end tail of the distribution, which in this case is the region of most interest. The quantile plot compares the inverse GEV, represented by the MLE of received power w (5), with the empirical data. Therefore, the quantile plot consists of the points:
(9)
where from (5):
(10)
A quantile plot which consists of points close to the unit diagonal indicates a good fit between the empirical and fitted distribution functions and any deviations from linearity indicate a model failure. As shown in Figure 5, the empirical data is clearly linear following the unit diagonal very closely even up to the extreme values.
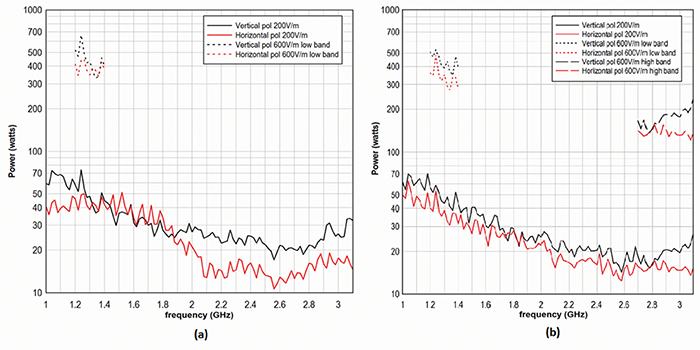
Another useful model diagnostic plot is the quantile estimation plot (commonly referred to as the return level plot in extreme value theory). This plot compares the predicted values from (5), which are based on the parameters estimates, with the empirical data wp, both plotted against yp on a logarithmic scale where yp = −ln(p)(over 0 < p < 1). More formally, the data points are:
(11)
and
(12)
This plot also provides insight into if the appropriate shape parameter was selected, in that the structure will be linear (for k = 0), convex (for k < 0) with a finite bound, or concave (for k > 0). Additionally, the variance of wp (7) can then be leveraged to provide 95% confidence intervals for the predicted values. By plotting the confidence intervals in the quantile estimation plot, a very good indication of distribution fitting is provided for the high end tail of the distribution due to the quantile scaling. Figure 6 shows the quantile estimation plot for the received power enclosure measurements. The plot depicts a very slight convex curvature indicating the Reverse Weibull distribution provides the best fit. However, the 95% confidence intervals are also depicted on the graph which indicates a linear (Gumbel) distribution is also possible.
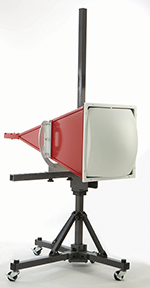
Conclusion
This application of the GEV distribution to model maximum received powers within a complex cavity was investigated. The GEV distribution provides three possible asymptotic maximum distributions, one of which is upper bounded, without requiring a priori knowledge of a parent distribution function. Experimental received power measurements were performed within an RF reflective cavity applying both mechanical and frequency stirring, as well as spatial sampling. Five hundred sample maximums were then statistically investigated and the Reverse Weibull representation of the GEV distribution was determined to be a good representation of the data. This aligns with the physically realistic situation, due to the fixed power density within the enclosure. However, parameter estimates and confidence intervals did not completely exclude the Gumbel distribution which aligns with the traditional exponential distribution function utilized in reverberation chamber theory for the overmoded case. Future measurements, specifically in the undermoded regime, are currently planned.
Acknowledgements
The authors gratefully acknowledge program support from Jeff Solka (NSWCDD IAR Program Lead) and Rachael Robinson (NAVSEA 05H/W CPSD Program Lead).
References
- D.A. Hill, Electromagnetic Fields in Cavities: Deterministic and Statistical Theories. New York: Wiley-IEEE Press, 2009.
- IEC 61000-4-21 Electromagnetic Compatibility: Reverberation Chamber Test Methods. Intern. Electrotech. Commission (IEC), Geneve, Switzerland, Aug. 2003.
- G.B. Tait and R.E. Richardson, “Wireless Channel Modeling of Multiply-Connected Reverberant Spaces: Application to Electromagnetic Compatibility Assessment,” IEEE Transactions on Electromagnetic Compatibility, Vol. 55, No. 6, pp. 1320-1327, Dec. 2013.
- D. Fedeli, G. Gradoni, V.M. Primiani, and F. Moglie, “Accurate analysis of reverberation field penetration into an equipment-level enclosure,” IEEE Trans. Electromagn. Compat., vol. 51, no. 2, pp. 170-180, May 2009.
- J.F. Dawson, T. Konefal, M.P. Robinson, A.C. Marvin, S.J. Porter, L.C. and Chirwa, “Field statistics in an enclosure with an aperture,” in Proc. 2005 IEEE International Symposium on EMC (Chicago, IL), Aug. 2005, pp.141-146.
- G. Orjubin, “Maximum Field inside a Reverberation Chamber Modeled by the Generalized Extreme Value Distribution,” IEEE Trans. On Electromagn. Compat., vol. 98, no. 1, pp. 104-113, Feb. 2007.
- S. Coles, An Introduction to Statistical Modeling of Extreme Values. Great Britain: Springer-Verlag London Limited, 2001.
Carl Hager is an electrical engineer with the Electromagnetic and Sensor Systems Department at the Naval Surface Warfare Center Dahlgren Division (NSWCDD). He can be reached at carl.hager@navy.mil.
Gregory Tait is an associate professor of physics at Bridgewater College, Bridgewater, VA. Dr. Tait has published over 90 papers in peer-reviewed professional journals and conference proceedings. He can be reached at vatais@verizon.net.